Separating the Signal from the Noise: The Limitations of Big Data
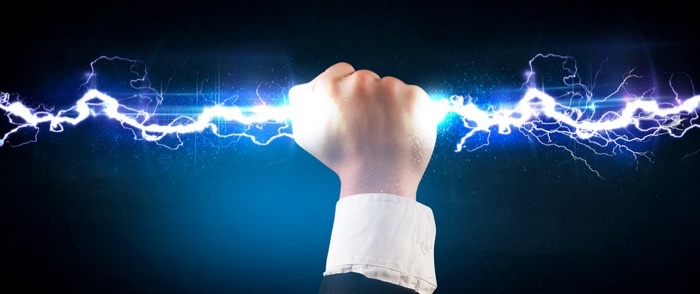
Predictive analytics tools put big data at your fingertips. Maximize the benefits and minimize the risks by thinking probabilistically.
In the world of big data, amidst a large and growing number of predictive analytics tools, it's increasingly common for consultants to offer services that offer to solve large problems connected to workforce management, performance, engagement, financial forecasting and more.
But in "The Signal and the Noise: Why So Many Predictions Fail—but Some Don't," Nate Silver asserts that data is useless without context. Silver himself claims to be an optimist when it comes to the potential of more data and more computing power to make us more productive. But he is equally concerned about the potential for error, inaccurate forecasts and predictions that are scales of magnitude from real-world outcomes.
"If you're using a biased instrument, it doesn't matter how many measurements you take," Silver says. One example might apply to engagement surveys. If survey administrators systematically underestimate the likelihood that employees will misrepresent their true feelings, more data won't improve the results of the survey.
No Context, No Chance
These observations raise significant warnings for experts using predictive analytics tools. Context is comprised by highly localized information. A standardized template may use a range of techniques that start to approximate real-world problems, but that fossilized template cannot replace the context of the problem being evaluated nor can it question its own assumptions in ways that might anticipate a significant break from the past.
Less Wrong, More Often
Big data creates as much noise as signal, which can make it harder to detect human biases that often hide amidst the assumptions. Silver points out that political pundits are rewarded more for dramatic predictions than for accuracy. Within organizations, financial forecasters can be tempted to agree with herd findings simply because such predictions are safer for career longevity.
Most people tend to mistake their successes as skill, while attributing failures to bad luck. Silver's insight is that this applies to all human endeavors. "Perhaps the central finding of behavioral economics is that most of us are overconfident when we make predictions," he argues.
According to Silver, a Duke University survey of corporate CFOs found that they "radically overestimated their ability to forecast the price of the S&P 500." One answer, he says, is to make more predictions and be rigorous in accounting for their accuracy over time.
Hedges for Better Predictions
Any given predictive analytics tool has the potential to bring powerful insights to your organization. Silver's argument is not to ignore big data, but rather be wary of the ways in which it can make us overconfident as we attempt to perceive the signal from the noise. "The goal of any predictive model is to capture as much signal as possible and as little noise as possible," he says. "Striking the right balance is not always so easy, and our ability to do so will be dictated by the strength of the theory and the quality and quantity of the data."
One common misperception is that agreement among forecasters amounts to accuracy. A better approach could be to encourage independent, nonreferential groups to tackle the same problem. The variance in findings can then become a strength, highlighting assumptions and potential errors in methodology and encouraging constructive dialogue.
Silver also makes an impassioned argument for the necessity of incorporating probabilistic predictions. He points out how fields like economics, finance and HR have been less rigorous about providing a margin of error. These groups may worry that such qualifications might weaken the perceived value of the finding. "They see uncertainty as the enemy — something that threatens their reputation," notes Silver. "They don't estimate it accurately, making assumptions that lower the amount of uncertainty in their forecast models but that don't improve their predictions in the real world."
The alternative is potentially devastating. Leaders may act on forecasts as if they were fact and in ways that can be devastating to your organization.